Anomaly Detection AI In Singapore
Anomaly Detection AI In Singapore
Anomaly detection Main article: Anomaly detection In data mining, anomaly detection, also known as outlier detection, is the identification of rare items, events or observations which raise suspicions by differing significantly from the majority of the data.[55] Typically, the anomalous items represent an issue such as bank fraud, a structural defect, medical problems or errors in a text. Anomalies are referred to as outliers, novelties, noise, deviations and exceptions.[56] In particular, in the context of abuse and network intrusion detection, the interesting objects are often not rare objects, but unexpected bursts of inactivity. This pattern does not adhere to the common statistical definition of an outlier as a rare object. Many outlier detection methods (in particular, unsupervised algorithms) will fail on such data unless aggregated appropriately. Instead, a cluster analysis algorithm may be able to detect the micro-clusters formed by these patterns.[57] Three broad categories of anomaly detection techniques exist.[58] Unsupervised anomaly detection techniques detect anomalies in an unlabeled test data set under the assumption that the majority of the instances in the data set are normal, by looking for instances that seem to fit the least to the remainder of the data set. Supervised anomaly detection techniques require a data set that has been labeled as "normal" and "abnormal" and involves training a classifier (the key difference to many other statistical classification problems is the inherently unbalanced nature of outlier detection). Semi-supervised anomaly detection techniques construct a model representing normal behavior from a given normal training data set and then test the likelihood of a test instance to be generated by the model.
Solutions
ARVAS - Anomaly Recognition Video Analytics System
Solutions | Anomaly Recognition Video Analytics System
Using machine learning and big data analysis, we enable a smarter, more powerful way of providing surveillance using ARVAS.
Traditional Surveillance Monitoring (lots of screens) to ARVAS innovative approach
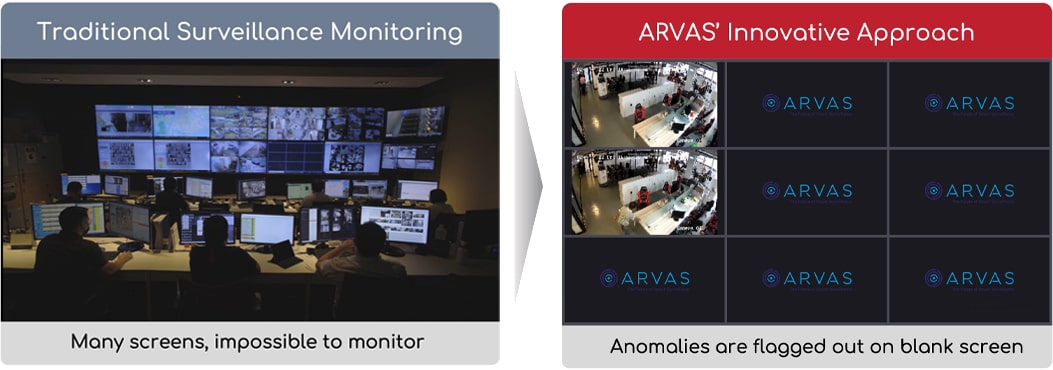
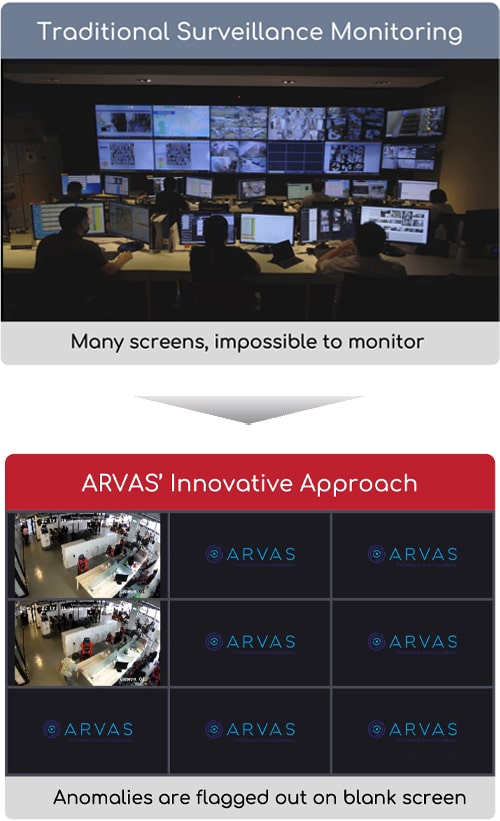
ARVAS Benefits
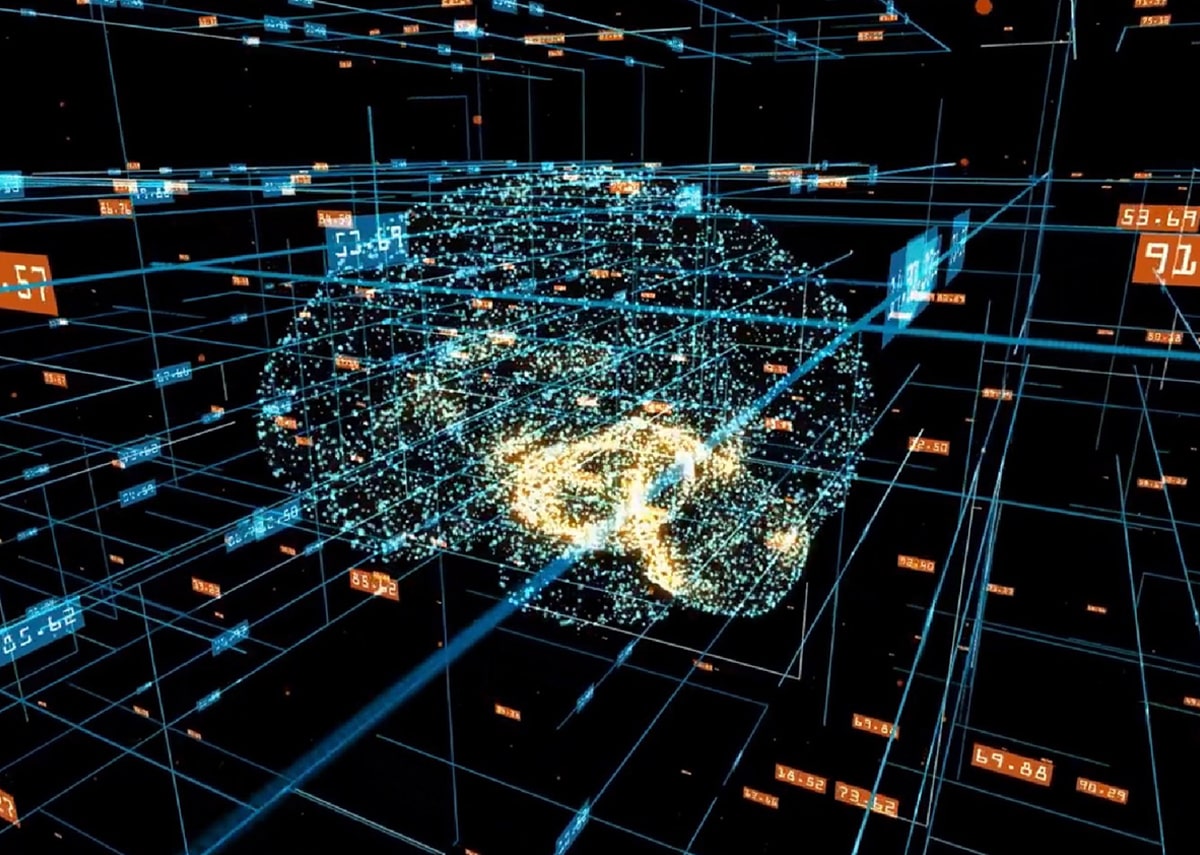
Self-Learning with
Wider Coverage
Unsupervised Machine Learning
ARVAS uses the latest machine learning techniques.
Autonomous Real-Time Detection
No set up of any rules needed, other than installation.
Unlimited Variation of Behaviours
Behaviours are no longer limited by fixed rules. ARVAS learns automatically to distinguish between normal and abnormal behaviours.
Easy Deployment
High Compatibility
Able to accept relatively low rates and resolutions, accommodating older legacy camera sources.
Not Scene Dependent
Applicable to any camera scene.
Highly Scalable
Scalable for thousands of cameras.
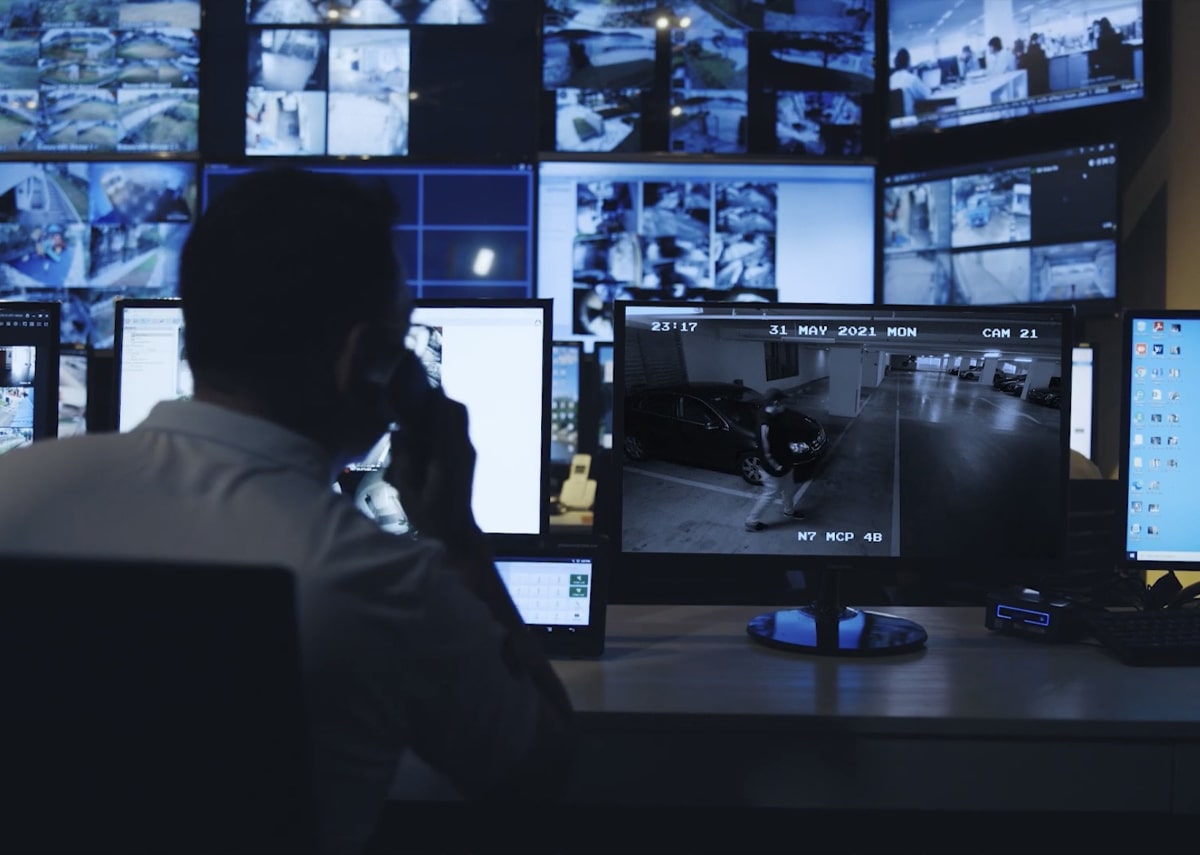
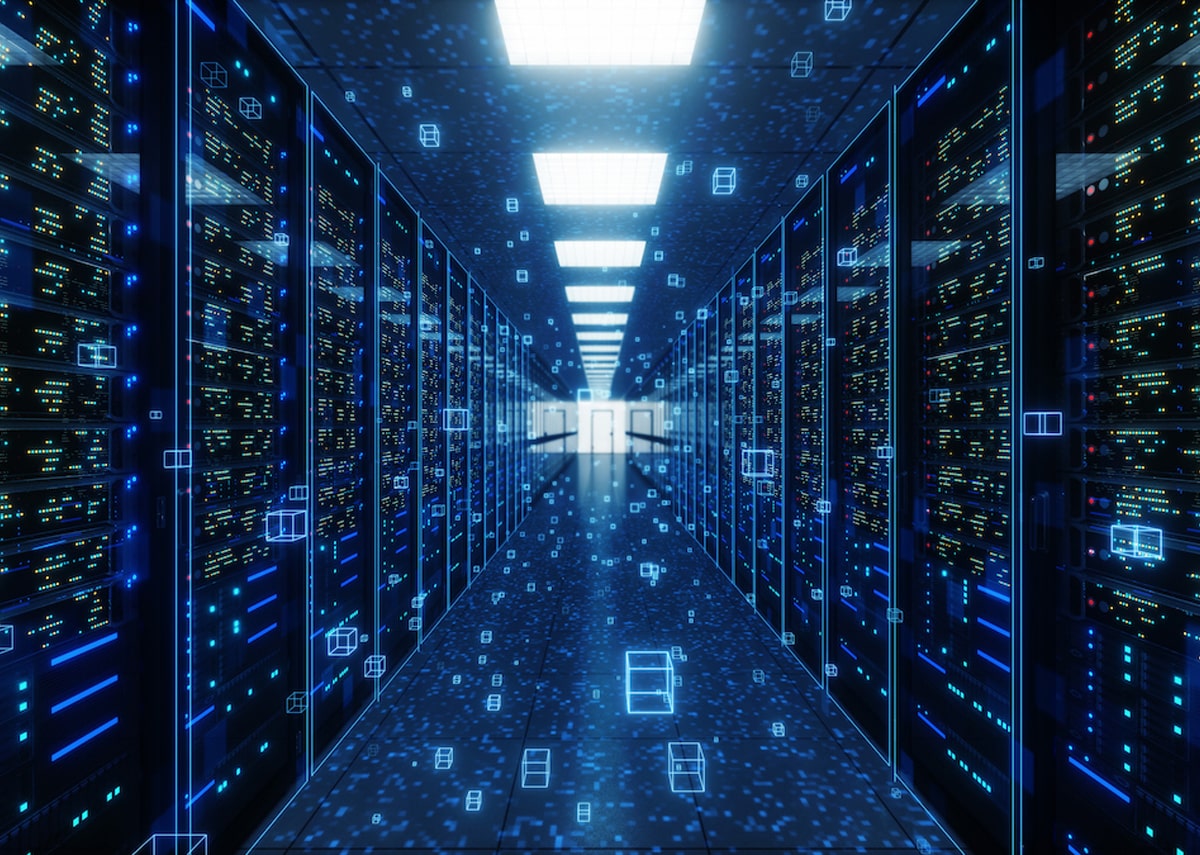
Highly Efficient and
Scalable Systems
Event Clustering
ARVAS’ system can cluster similar events together and allow user prioritization.
GPU Acceleration
Effectively serves a larger number of cameras per server. (Up to 200 cameras per server)
Effective, proactive monitoring leads to a lower reliance on routine physical patrols, especially during silent hours, leading to significant cost savings with improved security standards.
Detect Unlimited Range Of Anomalies With ARVAS
With self-learning capability, ARVAS can adapt to different environments and detect unlimited range of anomalies across all sectors
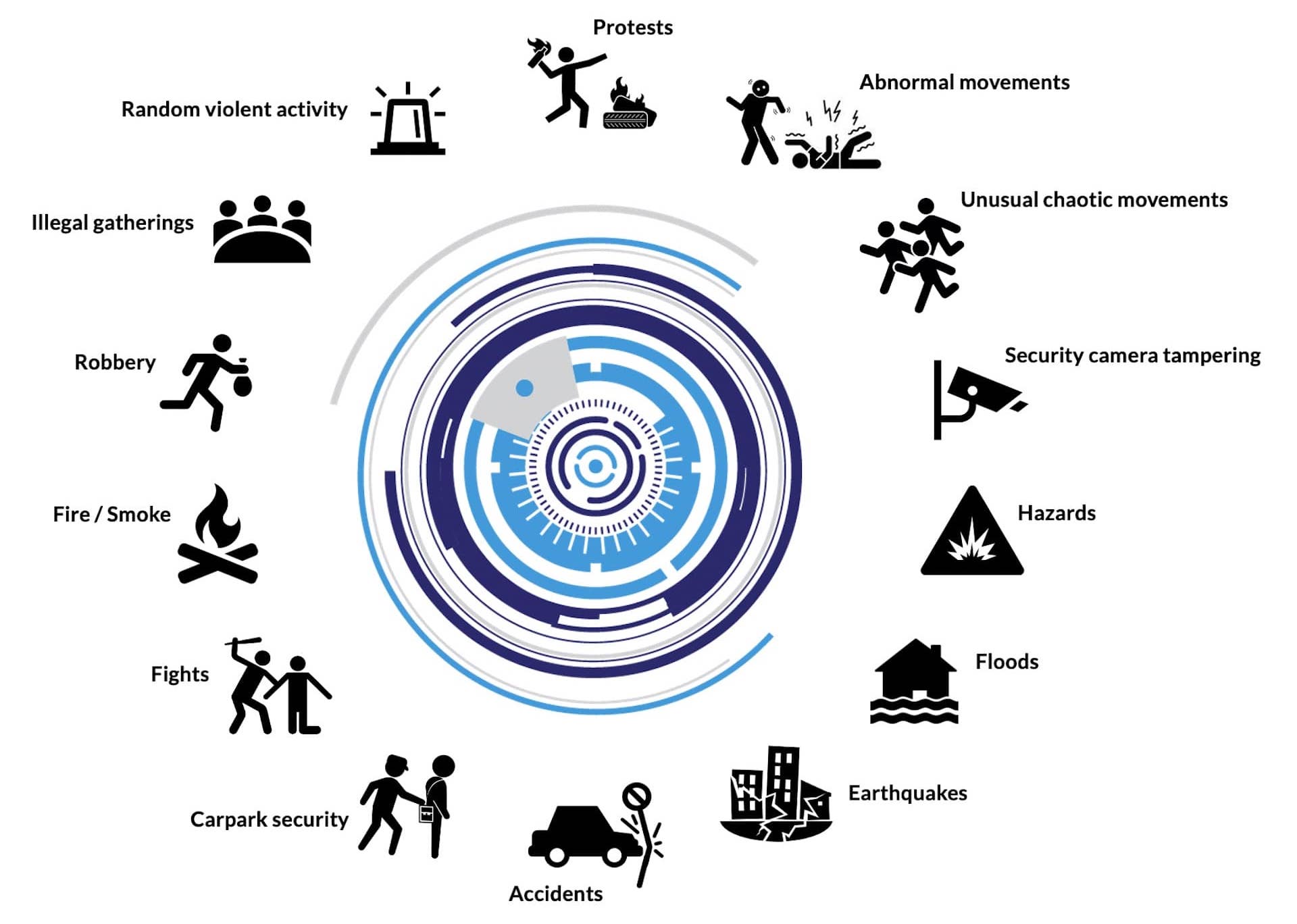
Hear from industry experts as they share how ARVAS is transforming the security industry.
ARVAS is integrated with these video management systems:
• Milestone
• Genetec
• NxWitness
• Huawei